Inside Nvidia’s Full AI Stack Stickiness
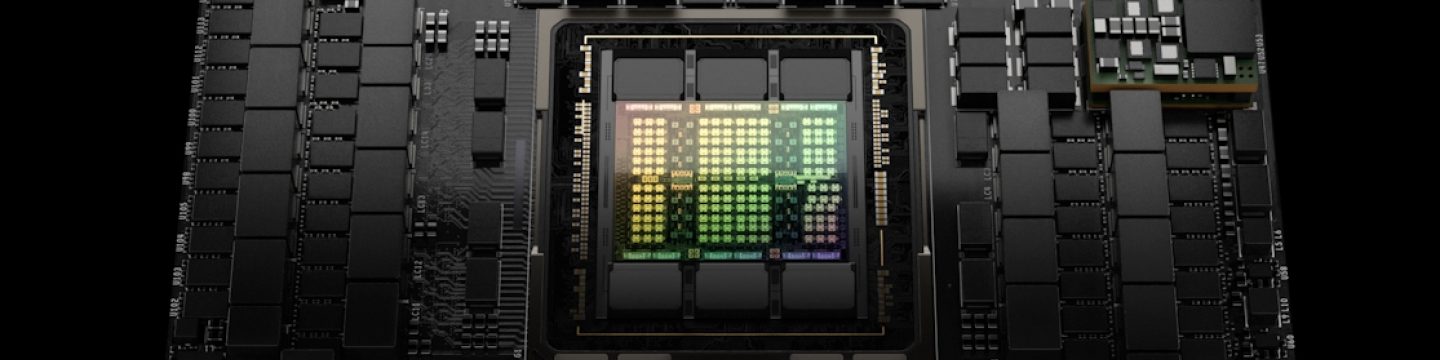
You couldn't miss the fanfare of NVIDIA’S massive earnings announcement, which blanketed the business press yesterday when the company announced a massive earnings beat as it exceeded revenue forecasts by $1.4 billion and forecast 170% annual growth for the next quarter.
The cloud infrastructure technology company’s blowout earnings and forecast for its fiscal second quarter was fueled by dominance in chips and systems for artificial intelligence (AI) infrastructure.
Some more numbers:
- Revenue of $13.5 billion was a new record, up 101% from a year ago.
- Data center revenue was $10.3 billion, up 171% from a year ago.
- Non-GAAP earnings were $2.70 per share, up 429% from a year ago.
Related Articles
Is CoreWeave Eyeing an IPO?
CoreWeave's advertising for an investor relations director and SEC expert. Could an IPO be in the works?
Lambda Scores $320 Million for AI Infrastructure CloudLambda, a cloud firm offering access to AI infrastructure via NVIDIA GPUs, has scored $320 million in Series C funding on a valuation of $1.5 billion
NVIDIA Unveils New GPUs, Networking, Enterprise toolsNVIDIA boosts its GPU position, improves its networking options, and serves up enterprise solutions. But it's still all about NVIDIA
The media at large loves to write about NVIDIA's dominance in graphics processing units (GPUs), the building blocks of AI workloads. But there's more. It's about the full stack.
As I wrote in today's column in Forbes, NVIDIA's rise to AI dominance involves the entire AI stack – from GPUs to optimized software libraries and specialized networking cards, including SmartNICs.
In the short term, NVIDIA's shares rose to an all-time high this morning, trading as high as $500, giving it a market cap of $1.2 trillion. Now, things seem a bit overheated and it wouldn't be surprising at all for investors to cash in some gains, but as we'll show, NVIDIA's market position is as strong as ever.
Going Viral with GenAI
NVIDIA's rise to dominance in AI over the past 10 years is an amazing story. But how it rode the viral generative AI wave over the past six months is even more amazing. Shares have gone from $115 to $500 in less than a year.
First of all: How did this happen? Less than a year ago, last December, investors were dumping shares of the largest cloud companies in the world, including Amazon, Facebook, Google, Microsoft, and Facebook, in fear of the looming cloud slowdown and even a possible recession.
Investors have already forgotten that NVIDIA shares crashed in 2022, falling from a high of $300 to a low of about $117. That’s right, I’ll break the news: You could have bought this $475 stock for as low as $117 in January!
In steps generative AI, the transformational technology that seemingly came out of nowhere but has been around for a decade or more. Generative AI went viral, promising to transform applications ranging from writing sales emails to faster drug discovery. The catalyst was the launch of OpenAI’s ChatGPT in November of 2022.
Large growth spurts are usually associated with big paradigm shifts – and it doesn’t get much bigger than AI. This year, generative AI went viral, sparking a new wave of tech investment that rescued the post-COVID lull.
But behind the massive AI hype is the reality that to create AI models and deploy them in applications requires a full-stack reworking of computing machinery. Traditional enterprise and cloud resources weren’t designed for generative AI.
From our Cloud Tracker Pro research service, Mary Jander outlined the rise of genAI in our "Navigating the AI Goldrush" report, listing the elements as below:
Accelerated computing. Generative AI modeling requires amounts of compute power generally classed as high performance computing (HPC). To offer customers this kind of processing heft, the hyperscalers are creating their own supercomputers. These facilities require accelerated computing, meaning the separation of computer parts that permits accelerator chips such as graphics processing units (GPUs) to offload data processing tasks from the central processing unit (CPU) of a server or servers. Cloud providers, including AWS and Google Cloud, have also come up with their own chips for AI processing. The hyperscalers are also creating alliances with NVIDIA and other suppliers to get the level of compute power they require to offer instances for generative AI modeling as part of their service repertoire.
High-speed networking among components. This is typically achieved via InfiniBand, but there are alternatives. NVIDIA recently announced Spectrum-X, a platform that augments high-speed Ethernet with specialized optics and other elements to provide a 400-Gb/s Ethernet link for use in AI clouds. And Broadcom in April announced the Jericho3-AI chip, which it claims will link 32,000 GPUs in a network with multiple ports running at 800 Gb/s. There is also a range of proprietary solutions from other suppliers, including Google, whose tensor processing units (TPUs) are interconnected with an optical circuit switch.
Expansion at the edge. While cloud vendors build up their supercomputing capabilities, they also must address edge resources to support generative AI applications. Once models are in place, smart cities, factories, healthcare agencies, etc., will require intelligence and processing power at the edge. The cloud titans continue to push out their networks worldwide.
NVIDIA Took a Decade to Prepare
NVIDIA plays the long game. Perhaps the best characteristic of NVIDIA is that it is a hardcore engineering company, with world-class R&D and M&A programs. NVIDIA is well-positioned for AI because it saw all this coming – and has been fortifying its portfolio and R&D for years.
I would argue that one of NVIDIA’s most important acquisitions over the past few years was Mellanox, acquired for $6.9 billion. Mellanox was also a hardcore network engineering company with Israeli roots. It also brought its own Ethernet switches and SmartNICs (network interface cards) to the party.
Specialized networking cards are crucial for AI workloads, which already must scale to 400 Gbit/sec to 800 Gbit/sec in throughput. Mellanox was also a dominant supplier of InfiniBand chips, a specialized networking technology for high-throughput server connectivity.
That's not to say other companies aren't enjoying the AI boom. In reporting its second quarter fiscal 2023 earnings earlier this month, Arista Networks reported 39% year-over-year growth, fueled by demand from hyperscalers such as Microsoft and Meta. One of its growth drivers is upgrades to higher bandwidth systems to drive AI workloads, said Arista executives.
Cisco also wants to get in on the party, though it’s a little late. Cisco said on its last earnings call that chips from its SiliconOne series are being tested by five of the six major cloud providers, without naming the firms. Testers include Amazon Web Services, Microsoft Azure, and Google Cloud.
Broadcom is also there. It announced the Jericho3-AI chip that can connect up to 32,000 GPU chips together, and it is a closely watched “AI play” among investors.
But NVIDIA’s integrated stack goes beyond those of dedicated networking companies. On the earnings conference call, CEO Jensen Huang talked about NVIDIA’s deep understanding of the software libraries needed to optimize AI workloads. This includes NVIDIA’s AI Enterprise software and CUDA platform, which includes acceleration libraries, pre-trained models, and APIs to enable development of applications.
And NVIDIA executives like to point out that the HGX system, basically “AI in a box,” includes 35,000 parts, 70 pounds, “nearly a trillion transistors, and advanced networking."
NVIDIA's earnings call was a seminal moment not just because it produced eye-popping numbers, but because it served as a demonstration of what a force the company has become through a methodical ten years of M&A, R&D, and deep technology integrations.
The Futuriom Take: The bottom line is that anybody that wants to play on the same level as NVIDIA in AI has a lot of pieces to put together. NVIDIA's achieved full-stack stickiness.
Related Articles
Is CoreWeave Eyeing an IPO?
CoreWeave's advertising for an investor relations director and SEC expert. Could an IPO be in the works?
Lambda Scores $320 Million for AI Infrastructure CloudLambda, a cloud firm offering access to AI infrastructure via NVIDIA GPUs, has scored $320 million in Series C funding on a valuation of $1.5 billion