Databricks/MIT Report Tells What's Lacking in Analytics
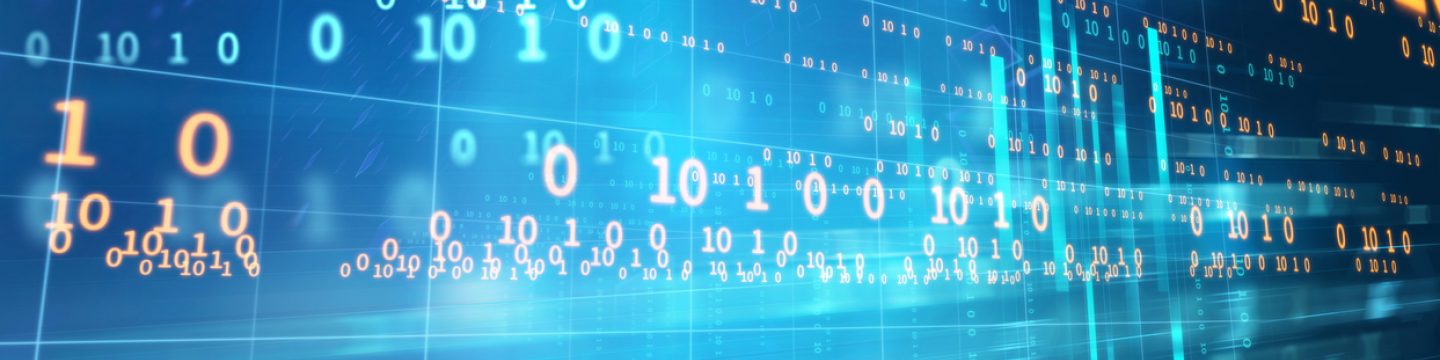
Data analytics and machine learning (ML) are top of mind for C-level IT professionals, who are struggling to establish relevant and valuable "data cultures" within their organizations.
That’s one of the key findings of a survey recently published by MIT Technology Review Insights and sponsored by Databricks. And of the 351 executive-level data professionals surveyed for the report, just 13% reported success in delivering an enterprise data strategy based on their companies' data.
The report, titled “Building a High-Performance Data and AI Organization,” cites a range of obstacles to achieving data success, including fragmented data sources, poorly performant data analysis tools, and limited communications with end users. Let’s take a closer look.
Enterprises Want Data Analytics and AI
Related Articles
Databricks' AI Ambitions Revive IPO Chatter
Databricks' large language model DBRX was recently released to general applause. Could it be another step toward IPO?
Directus Takes Aim at Database DemocracyDirectus, a small firm from Brooklyn, N.Y., has revamped its data management platform to unify SQL data from multiple databases without changing underlying schema or content
The Top 10 Cloud Tech Trends of 2021Futuriom takes a look back at the top trends in cloud technology for the year 2021 and peeks at what's ahead
According to the MIT/Databricks survey, improving data quality and processing is the most important enterprise-wide data strategy initiative for the next two years, as reported by 48% of respondents (presumably, 51% have more specific projects underway for their data). Forty-three percent also want to increase adoption of cloud platforms; 43% look to enhance data analytics; 42% want to enhance their applications of ML; and 38% are seeking to expand usage of all data in their organizations.
Of course, the road to data analytics and AI is clearly through the cloud, which is the enabler of data “lakehousing” (a Databricks term) that enables structured, semi-structured, and unstructured data to be organized in a common location for ML, analytics, and efficient querying across multi-cloud networks.
That sounds great, but most organizations surveyed report falling short of the ideal. On a scale of 1 – 10, with 10 signifying success, just a handful (13%) of respondents rate themselves a 10 in meeting their data goals. As shown in the chart below, a full 75% think their success rate falls between 7 and 8, and 12% believe their performance rates a 4 to 6. While no one admitted to failing to meet goals, the data says that 87% think they could do better.
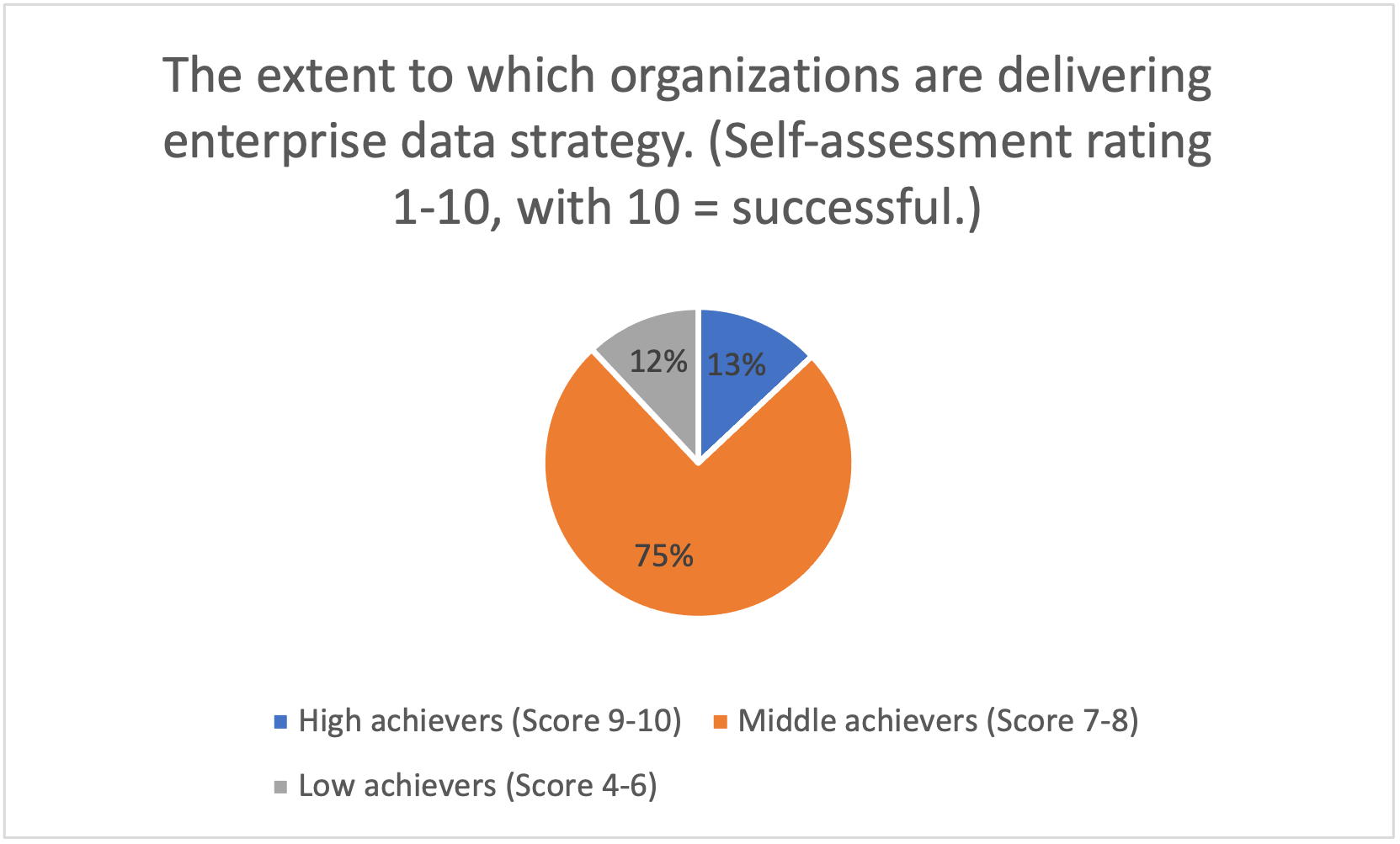
Source: “Building a High-Performance Data and AI Organization,” MIT Technology Review Insights.
What’s Getting in the Way of Data Analytics/AI
Of the respondents acknowledging their status as low achievers on the data delivery scale, a full 44% said their data platform does not easily scale; 39% reported slow processing of large amounts of data; 22% reported high data duplication; and 20% said the tools they use for ML are complex and fragmented.
As a result of these shortfalls, 50% of survey respondents reported that they're evaluating or implementing new data platforms. Of those seekers, 50% are looking for open-source standards and open data formats as a means of reducing the need to switch vendors when products are mixed, matched, and upgraded; 49% want better security and governance for data; 44% want better price/performance for data infrastructure, operations, and maintenance; and 41% want to support a variety of analytics use cases, not just one such as ML.
Non-Technical Issues Surface in ML
While using data lakes and warehousing technologies appear to boost analytics efficiency and enable ML, organizational challenges can throw a wrench in the works, according to the survey. Among the low-achieving data managers surveyed, 29% said it was difficult for teams with differing or “cross-functional” mandates to collaborate on analytics use cases.
“If your machine learning use case isn’t mapping very closely to a business use case, then I’m wondering what you’re doing with it,” said Andy McQuarrie, CTO at Hivery, an Australian firm specializing in retail AI, who was interviewed for the report.
The survey concludes that simplifying the data analytics/AI process and making it accessible and meaningful to a variety of users, not just engineers, is essential to creating a so-called data culture. In another quote in the report, Patrick Baginski, senior director data science at fast-food titan McDonald’s sums it up: “The quicker you can demonstrate value from ML and data science, the quicker you in get users’ buy-in and build management confidence in the value of both to the organization.”
Related Articles
Databricks' AI Ambitions Revive IPO Chatter
Databricks' large language model DBRX was recently released to general applause. Could it be another step toward IPO?
How Telcos Are Prepping for Generative AICommunications service providers have traditionally been slow on the uptake when it comes to new technologies. But they are prepping for generative AI
What's Up with the Change Healthcare Attack?A ransomware attack at a major U.S. healthcare service provider highlights the need for better protection driven by AI