What Is Edge Computing for IoT?
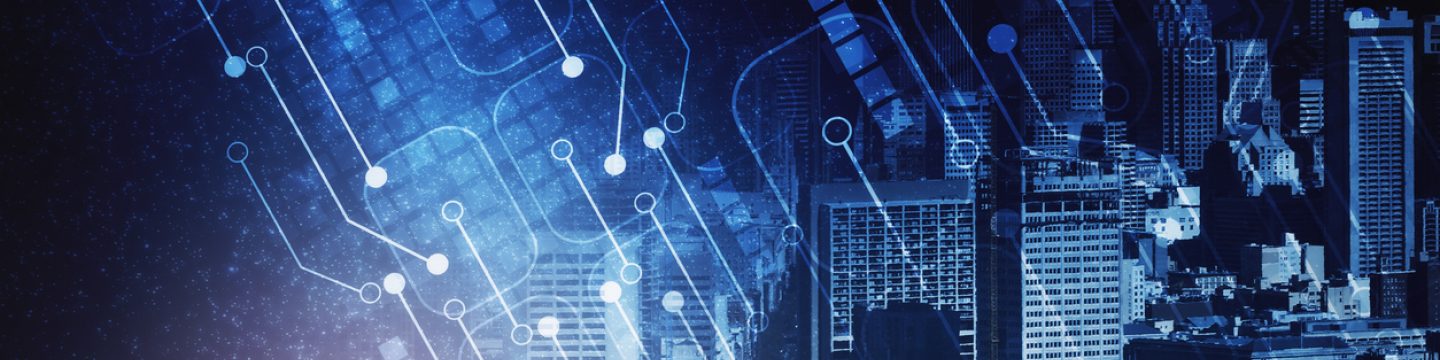
There is an excessive amount of data generated by a growing number of "connected" devices, and this will continue as Internet of Things (IoT) technologies and use cases evolve in upcoming years. As per research firm Gartner, by 2020 there will be as many as 20 billion connected devices producing gigabytes of data per user. These devices will not be just smartphones or laptops but will include connected cars, vending machines, smart wearables, medical robots for surgery, and many more.
A massive amount of data generated by myriad types of such devices needs to push to a centralized cloud for preserving (data management), analysis, and decision making. Resultant analytics data then gets transported back to devices. This round trip of data consumes network infrastructure as well as cloud infrastructure, which can further lead to lower latency and bandwidth issues, affecting mission-critical IoT use cases. For example, in self-driving connected cars, a high volume of data is generated per hour; that data has to upload to the cloud, be analyzed, and result in instructions sent back to the car. Low latency or resource congestion may delay a response to a car, which could result in an accident.
Edge Computing for IoT
This is where edge computing comes into the picture. An edge compute architecture can be used to optimize cloud computing systems for performing data processing and analytics at the edge of network, closer to the source of data. With this approach, data is collected and processed near the device itself, rather than sending it to cloud or data center.
Edge computing benefits:
- Edge computing can decrease the network bandwidth (i.e. lower latency) required between sensors and central cloud and reduce a burden on whole IT infrastructure.
- Data is stored and processed at the edge devices without requiring network connectivity for computation provided by the cloud. That eliminates high bandwidth continuous network connection.
- With edge computing, endpoint devices send only information that is needed for cloud computing instead of raw data. It helps to cut costs for connectivity and redundant resources at cloud infrastructure. This is beneficial when massive data produced by industrial machinery is analyzed at the edge and only filtered data is pushed to the cloud, leading to a significant saving in IT infrastructure.
- Utilization of computational power leads edge devices to behave like cloud-like operations. Applications can be executed rapidly and create robust and highly responsive communication with an endpoint.
- Security and privacy of data are achieved with edge computing: Sensitive data is generated, processed, and kept at the edge device, rather than being transferred over the unsecured network and risking a breach on the centralized data center. Edge computing ecosystems could have common policies (which can be implemented in an automated way) for each edge for data integrity and privacy.
The emergence of edge computing does not replace the need for traditional data center or cloud computing infrastructure. Instead, it coexists with the cloud as computing capabilities of the cloud are distributed to endpoints.
Machine Learning at the Network Edge
Machine learning (ML) is complementary technology to edge computing. In machine learning, generated data is fed to the ML system to produce analytics decisions models. In an IoT and edge computing scenario, machine learning can be implemented with two approaches. The first: ML algorithms require huge computational power to produce decisions only possible in the cloud. Data gathered from edges will be fed to ML systems, where a learned analytics decision model is produced and then pushed to edge of the network. In this way, analytics decisions can be made at all edge devices. In this model, edge devices will be used to collect, analyze, and take actions in the cloud, enhancing intelligence.
The second method: If endpoint devices send sensor-generated data to ML systems in the cloud, a significant amount of time will be spent on transporting and processing data by ML systems for producing analytics decisions. For this, smart machine learning or artificial intelligence (AI) chips can be introduced, and endpoint devices will send data to the cloud for storage purpose only. It takes less computing power to enable machine learning capabilities at the edge of the network.
Edge Compute & IoT Conclusions
Edge computing, along with machine learning technology, provides IoT an advantage for a future surge towards agile communications. The upcoming 5G telecom network will offer a much more advanced network for IoT use cases. Along with high-speed low-latency data transfer, 5G will offer a Mobile Edge Computing (MEC)-based telecom network, enabling automated implementation and deployment of edge services and resources. Moving further in this revolution, IoT device manufacturers and software application developers will be eager to take advantage of edge computing and analytics. We will see more intelligent IoT use cases along with an increase in intelligent edge devices.