Scale AI Scores $325 Million to Grow AI Solution
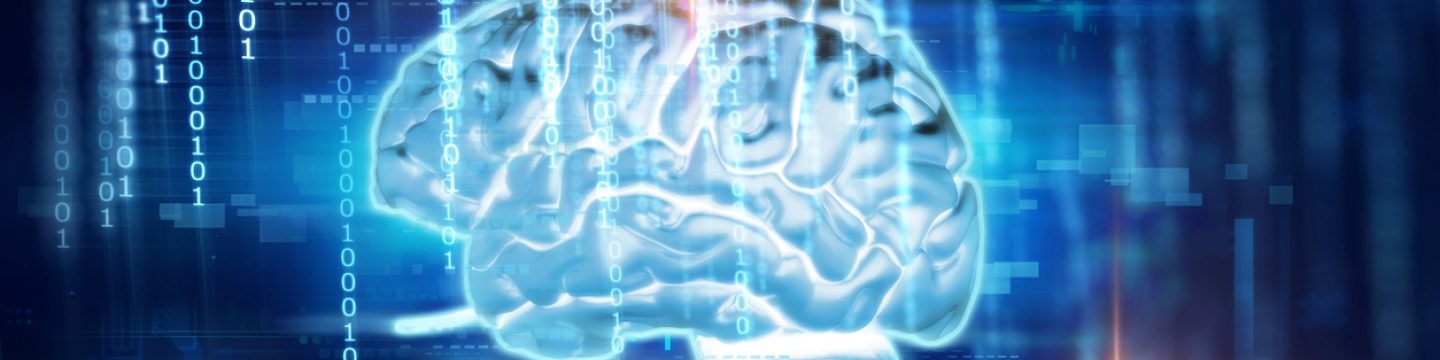
Scale AI, a late-stage startup based in San Francisco, has scored $325 million in Series E funding at a valuation that tops $7 billion. And just as newsworthy as the money is what Scale’s done to get it—namely, alleviate a major pain point for enterprise use of artificial intelligence (AI) and machine learning (ML).
Founded in 2016 by MIT dropout and ex-Quora tech lead Alexandr Wang, Scale AI has tracked impressive growth. In less than two years, it’s garnered $580 million in funding, bringing its total to just over $600 million.
Its investors could fill a city bus, but the latest round was co-led by Dragoneer, Greenoaks Capital, and Tiger Global, with participation from Wellington Management and Durable Capital, along with existing investors Coatue, Index, Founders Fund, and YC. Accel Partners, Thrive Capital, Spark Capital, and a range of individuals are among investors in past rounds.
Scale AI claims to have “hundreds” of customers, including Brex, DoorDash, Etsy, General Motors, iRobot, NVIDIA, Oshkosh, PayPal, Pinterest, Samsung, SAP, Square, Toyota, the U.S. Department of Defense, and the U.S. Air Force. It claims 489 employees. It has its own conference.
Scale AI Picked a Hot Spot
What’s this startup doing right? Scale AI’s success comes from meeting a significant need among the growing number of enterprises looking to leverage AI and ML to automate processes and boost business. Scale AI does this by handling the data preparation for ML modeling that otherwise relies heavily on manual work by specialist firms. There’s plenty of help that way, but it can be slow and costly.
To back up a bit: Machine learning uses input data iteratively to discern and predict statistically-specified outcomes. The challenge is to feed ML models what they need in order to derive the right results. Without some form of automated labeling, getting databases that are overflowing with information into a model to answer specific questions can be daunting. That’s the problem Scale AI addresses. And it is fundamental to the creation of machine-learning models that are increasingly used to solve problems and automate processes in a range of vertical markets.
Scale AI’s solution is a series of application programming interfaces (APIs) that ingest raw data to a proprietary platform and use a combination of Scale AI’s own technologies, human expertise, and machine learning to label and annotate the data so that it can then be used for ML modeling.
Human labeling isn’t eliminated, but it’s significantly reduced, and the margin of human error narrowed.
Scale AI’s Focus
Scale AI has succeeded in part by focusing on a specific solution that's in demand. It has also narrowed its focus to specific markets where machine learning and AI are becoming essential to business. These include autonomous vehicles, government, document management, retail and e-commerce, and robotics and manufacturing.
Scale AI also has picked its next problem to solve — managing the AI development cycle. A product called Nucleus was released last year that purports to marshal the process of creating ML models with increased efficiency and success. The product had its origins in Helia.ai, a company that specialized in computer vision software for video analytics, whose staff Scale AI acquired in November 2020 (terms undisclosed). Co-founder and former Helia CEO Russell Kaplan (ex-Tesla) now heads up the Nucleus team at Scale AI.
ML Labeling Competition On the Rise
Scale AI has drawn ahead of a growing cohort of startups in an increasingly crowded field that are seeking to till the fertile area of automated data prep for ML. To name just two: Labelbox, founded in 2018 in San Francisco, which has raised $79 million from Andreessen Horowitz and Kleiner Perkins, among others; and Hive, a company founded in 2013 and backed by General Catalyst and Founders Fund.
Computer vision modeling is a particularly popular niche for ML labeling, the kind used to track pedestrian traffic at the mall or to create smart traffic-monitoring cameras, for instance. An Open Source Computer Vision Library (OpenCV) is available that offers open source computer vision and machine learning software, including help with annotation. Clarifai, founded in 2013 and headquartered in Delaware, specializes in computer vision ML and has gotten $40 million from Union Square Ventures and Menlo Ventures, among others.
Larger companies also see opportunity in this niche, and all three cloud leaders offer APIs that streamline machine learning and in particular computer vision ML models. Microsoft (MSFT) offers a Computer Vision API and Video API as part of Azure Computer Vision and Azure Custom Vision. Google offers similar service as part of Google Cloud Platform.
Expect these kinds of tools to proliferate as applications speed up with 5G, particularly at the network edge. The world of IoT and industrial IoT will be particularly rife with automated solutions — and the machine learning models that help to create them.