NVIDIA Unveils New GPUs, Networking, Enterprise tools
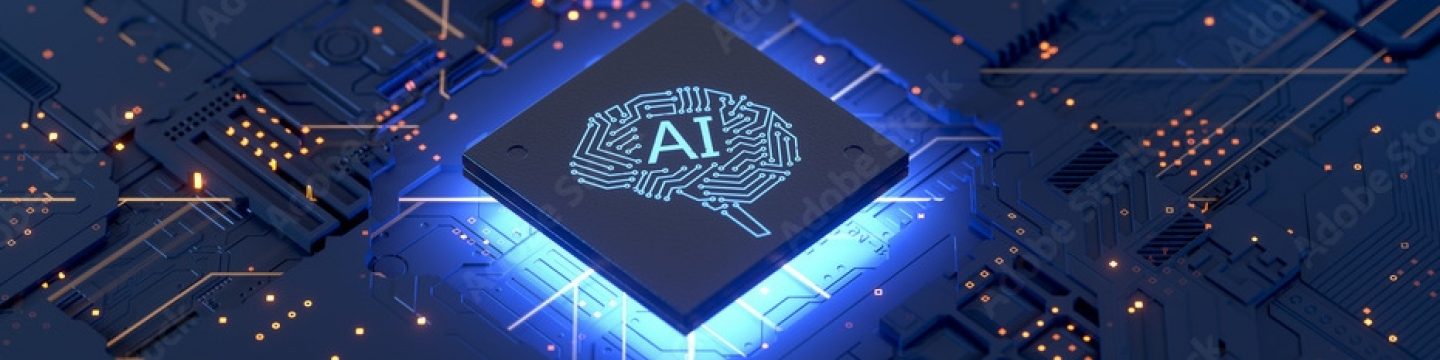
NVIDIA released an array of new accelerated computing platforms, AI networking products, and tools and APIs for enterprise AI development – all while maintaining its tight grip on proprietary hardware and software.
“Accelerated computing has reached the tipping point,” said NVIDIA founder and CEO Jensen Huang in a lengthy keynote at the vendor's GTC conference in San Jose, Calif., on Monday, March 18. “General purpose computing has run out of steam. We need another way of doing computing…”
As AI models get larger and more complex, it can take up to 30 billion petaFLOPS of compute power to train them. This has led NVIDIA to advance beyond its Hopper GPU series to a new computational platform for AI named Blackwell. NVIDIA says the Blackwell GPU is “built to democratize trillion-parameter AI,” becoming the “engine of the new industrial revolution” and offering up to 20 petaFLOPS of performance per chip. This represents a performance improvement of up to 4 times for training and 30 times for inferencing – while supporting 25 times better energy efficiency than the top end of the Hopper GPU series.
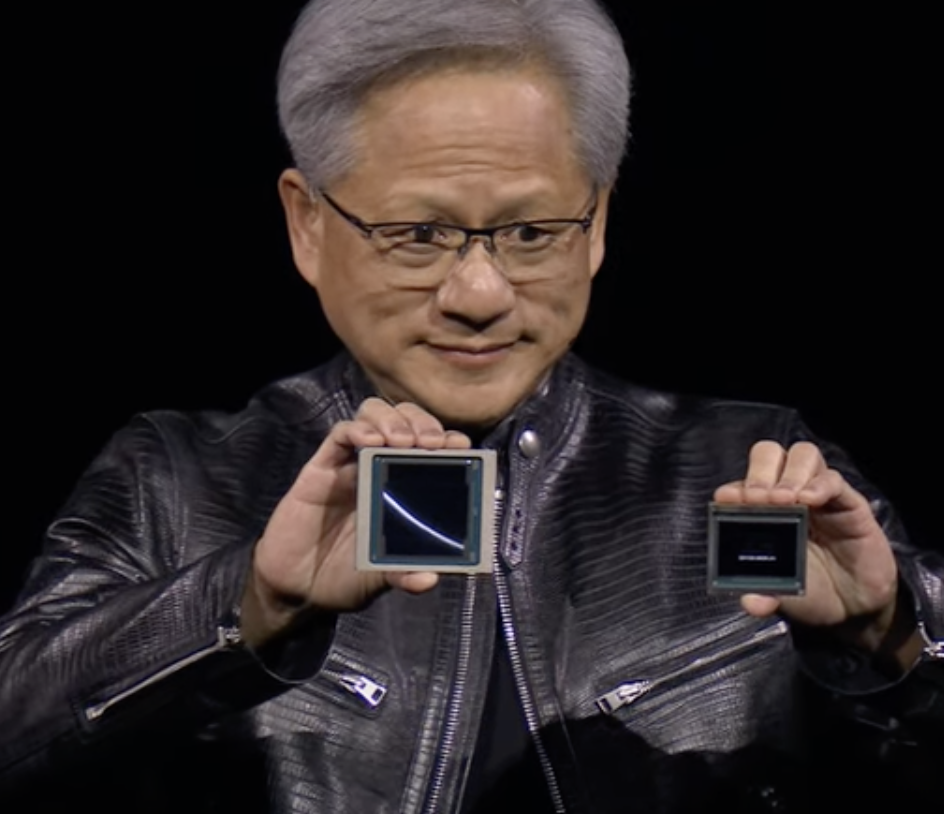
Related Articles
Arrcus Lands $30 Million with Help from NVIDIA
MCN software player Arrcus scores significant investment from NVIDIA, which is also collaborating with Arrcus on AI networking solutions
Can NVIDIA Keep Up with Itself?NVIDIA reported record earnings again last night. But supply isn't meeting demand, and the competition is growing
NVIDIA Takes AI Supercomputer to the CloudNVIDIA unveils a suite of AI components, including ones that power a supercomputer service offered through Oracle Cloud Infrastructure and other clouds
NVIDIA CEO Jensen Huang compares Blackwell and Grace chips during recent keynote. Source: NVIDIA
A combination of two Blackwell GPUs and one Grace CPU is used to create the GB200 Superchip, a fundamental building block for generative AI training that supports 40 petaFLOPS of AI performance. A Blackwell compute node comprised of four Blackwell GPUs and four Grace CPUs delivers 80 petaFLOPS of power. The GPUs are connected via a ConnectX-800G InfiniBand SuperNIC. A Bluefield-3 DPU provides line-speed processing of networking, storage, and security, supporting computing within the network and providing 80 Gb/s of bandwidth.
The message of all this? The scale just keeps growing. A new platform called the GB200 NVL72 packs 72 Blackwell GPUs and 36 Grace CPUs connected by NVLink technology in a single rack, supporting 720 petaFLOPS of training horsepower. The fifth generation of the internal chip-to-chip networking technology NVLink supports up to 1.8 TB/s bidirectional throughput per GPU for up to 576 interconnected GPUs.
The setup will be connected to the DGX Cloud. It is fully liquid cooled.
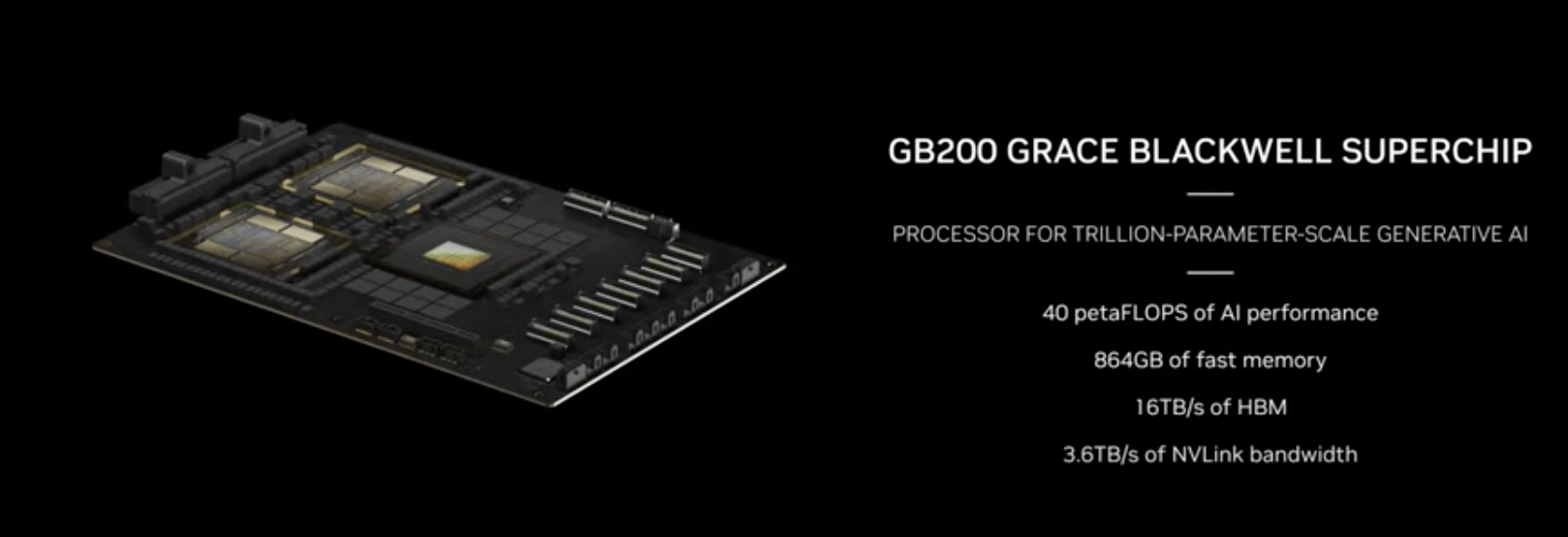
NVIDIA GB200. Source: NVIDIA
“This will expand AI datacenter scale to beyond one hundred thousand GPUs,” said Ian Buck, VP, Hyperscaler and HPC, on a briefing call with analysts.
Networking: Not Just InfiniBand
To get the most benefit from the new NVIDIA chips requires associated networking, which raises the issue facing many enterprises today: In selecting an AI environment, should they stick with NVIDIA’s proprietary InfiniBand connectivity solution or go with a version of Ethernet designed for AI? After all, the world’s leading switching suppliers, including Arista, Broadcom, Cisco, and Juniper have joined the Ultra Ethernet Consortium, which appears to be gaining some momentum as a proposed alternative to InfiniBand. Notably, so far each of these vendors has its own take on how to improve Ethernet to support full utilization of GPU resources. What the consortium produces will likely reflect some of these innovations.
NVIDIA is hedging its bets by playing at both ends. To interconnect multiple GB200s, customers have two connectivity options: the NVIDIA Quantum-X800 InfiniBand and the NVIDIA Spectum-X800 Ethernet switches. The Quantum-X800 links to the NVIDIA ConnectX-8 SuperNIC to support up to 800-Gb/s end-to-end InfiniBand throughput; the Spectrum-X800 works with the NVIDIA Bluefield-3 SuperNIC to support the same rate.
CEO Huang and other execs remain silent on the issue of either/or in networking, perhaps waiting to see what the market decides. So far, the hyperscalers appear to favor both. NVIDIA says Microsoft Azure, Oracle Cloud Infrastructure, and CoreWeave are among early adopters of both NVIDIA switching alternatives.
What About the Enterprise?
NVIDIA has become the premier supplier to the world’s largest implementers of AI training, those companies such as Meta, AWS, Microsoft, Google, and Oracle that require enormous compute resources for training large language models (LLMs). But implementation of AI will happen at the enterprise level, where companies will feed their data into these models -- at the inferencing stage -- to create applications.
Enter NIMs, short for NVIDIA Inference Microservices, described as follows by a group of NVIDIA technologists in a recent blog:
“NIM is a set of optimized cloud-native microservices designed to shorten time-to-market and simplify deployment of generative AI models anywhere, across cloud, data center, and GPU-accelerated workstations.”
NIMs are packaged to run on NVIDIA infrastructure, incorporating the CUDA platform along with the NVIDIA Triton Inference Server and other elements required for inferencing at the enterprise level. NVIDIA has worked with SAP, Adobe, ServiceNow, Cadence, and Crowdstrike to create NIMs that will act as application platforms. Cohesity and Dropbox NIMs are reportedly in the works. Multiple NIMs can be deployed for each application.
Reliance on CUDA is significant here. Also, it’s important to note that customers will need to use the application programming interfaces (APIs) associated with each NIM to build applications based on specific models. In the fine print, NIMs provide a range of advantages to enterprise customers, but their use depends on NVIDIA technologies.
It’s Still All About NVIDIA
This week’s announcements underscore NVIDIA’s position as keeper of the AI keys.
“We see and we believe that the NVIDIA GPU is the best place to run inference on these models,” said Manuvir Das, VP of Enterprise Computing at NVIDIA, in the analyst briefing mentioned earlier. “We believe that NVIDIA NIM is the best software package and the best runtime for developers to build on top of so they can focus on enterprise applications and just let NVIDIA do the work…”
Futuriom Take: NVIDIA’s GPUs and associated technologies retain a tight grip on the market. While supplying enterprises in a range of vertical markets with the tools needed to create AI apps, it’s clear that much of the work will require NVIDIA technologies, which will remain ascendant for the near term.
Related Articles
NVIDIA Leads Trend in Generative AI
NVIDIA's CEO Jensen Huang is delivering the message: NVIDIA is leading the charge in the twin trends of accelerated computing and generative AI
Can NVIDIA Keep Up with Itself?NVIDIA reported record earnings again last night. But supply isn't meeting demand, and the competition is growing
NVIDIA Takes AI Supercomputer to the CloudNVIDIA unveils a suite of AI components, including ones that power a supercomputer service offered through Oracle Cloud Infrastructure and other clouds