Google Says Its AI Accelerator Outperforms NVIDIA's
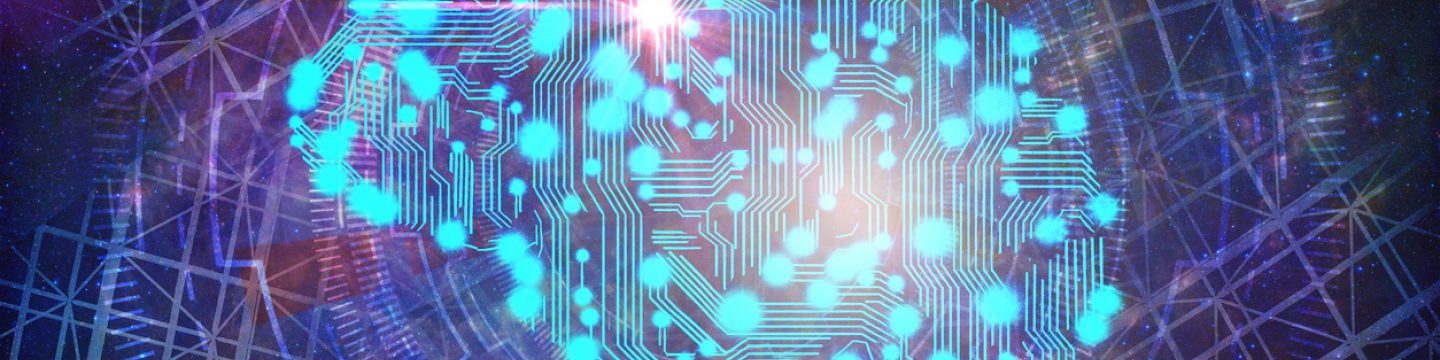
In the latest sprint in the artificial intelligence (AI) chip race, Alphabet’s Google says its AI-specific TPU v4 supercomputer outperforms its predecessor by an order of magnitude while being faster and more energy efficient than solutions from Graphcore and NVIDIA (Nasdaq: NVDA).
A recent blog post by Google Fellow Norm Jouppi and Google Distinguished Engineer David Patterson states that the TPU v4 (TPU being short for Tensor Processing Unit) is capable of performing machine learning (ML) tasks ten times faster than the vendor’s TPU v3. A recent scientific paper by the two and numerous colleagues also states:
“For similar sized systems, [the TPU v4] is ~4.3x-4.5x faster than the Graphcore IPU Bow and is 1.2x-1.7x faster and uses 1.3x-1.9x less power than the Nvidia A100.”
The Need for Speed
Google's claims for top-speed AI processing are significant because the blast of AI activity in today's market demands compute power unheard-of even several years ago. To create AI engines such as Google's Bard or OpenAI's ChatGPT calls for training models that crunch massive amounts of data to identify patterns that translate to coherent functions.
The process involves large language models that use complex algorithms to identify those patterns in data. These models are used for more than chatbots. They also fuel computer vision applications and AI recommendation systems for healthcare and software development.
So What's the TPU v4?
The TPU v4 isn’t a chip by itself but is instead a platform comprising multiple pods, each containing 4,096 chips, interconnected by an optical circuit switch (OCS) developed by Google. The result is a massive configuration of processing power required to train AI applications to perform as directed.
Google made TPU v4 slices available to Google Cloud customers in May 2022. The vendor revealed at the time that it was using TPU v4 technology to power a massive Google Cloud system in Mayes County, Oklahoma. Google boasted that the system offered 9 exaflops of aggregated compute power (the equivalent of roughly 90 million laptops). It also claimed to be running on 90% carbon-free energy.
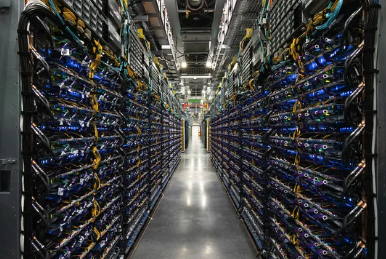
Google Cloud TPU v4 supercomputer system. Source: Google
Key to the performance of the TPU v4 is its OCS, which was developed to be dynamically reconfigurable, Google claims. In their blog post, Jouppi and Patterson state:
“TPU v4 is the first supercomputer to deploy a reconfigurable OCS. OCSes dynamically reconfigure their interconnect topology to improve scale, availability, utilization, modularity, deployment, security, power, and performance. Much cheaper, lower power, and faster than Infiniband, OCSes and underlying optical components are <5% of TPU v4’s system cost and <5% of system power.”
What's It Mean to NVIDIA?
Google’s claims to be faster and more efficient than other chips hit squarely at NVIDIA, which has a leading role in the AI chip race. NVIDIA isn't having it.
NVIDIA notes that the A100 to which Google compares the TPU v4 has been superseded by NVIDIA’s H100, which is based on NVIDIA’s new Grace Hopper architecture. NVIDIA says the H100 speeds up large language models by 30X compared to the H100. Further, the H100 comes not just with InfiniBand interconnect capabilities but optionally with the NVIDIA NVLink Switch System geared for higher-end AI applications. NVIDIA claims the H100 with NVLink performs AI training 9X faster than the A100.
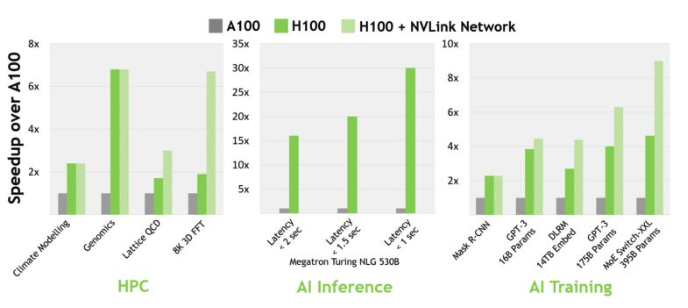
NVIDIA compares its A100 to H100. Source: NVIDIA
An NVIDIA spokesperson on April 5 sent this statement to Futuriom:
"NVIDIA this morning released its latest MLPerf Inference results, the industry’s only independent, third-party standard for measuring AI performance.
"The new MLPerf Inference v3.0 benchmark results show NVIDIA’s current generation H100 delivers the highest AI performance across seven categories including natural language understanding, recommender systems, computer vision, speech AI and medical imaging."
For its part, Graphcore told Futuriom in an email: "It’s really hard to offer a meaningful comment as we’ve never submitted to MlPerf inference – so haven’t tested IPUs under the same conditions on the same model."
There will likely be many more claims for AI accelerators as the market builds. Companies large and small are shouldering in for a place at the AI table. Gauging by the array of applications requiring AI, there will probably be room for many.