What's Next for Networking Infrastructure for AI?
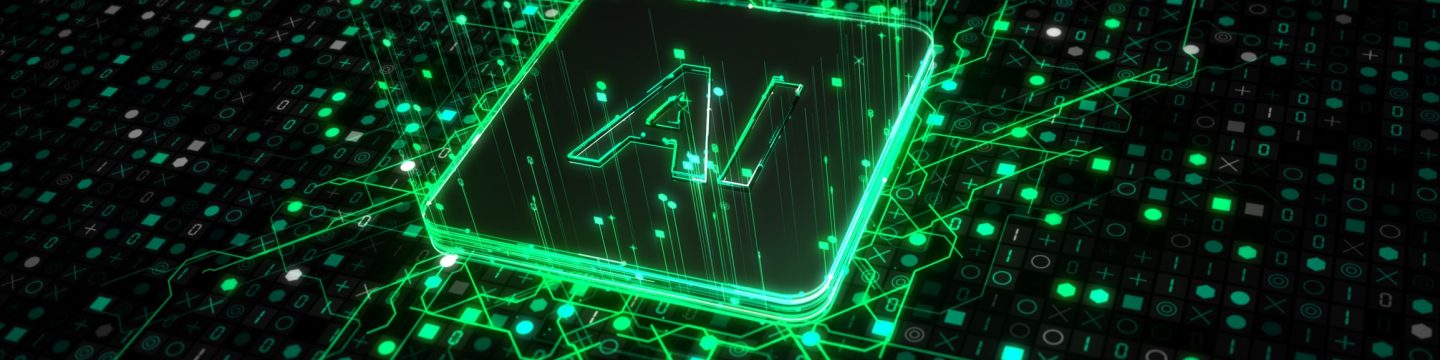
Artificial intelligence (AI) is building momentum in enterprises worldwide. Futuriom's research shows that hundreds of the globe's largest firms are engaged in proof-of-concepts to bring AI to bear on a range of critical applications. At the same time, to reap AI’s rewards requires a full-on reworking of the IT infrastructure, with particular emphasis on networking.
It’s clear why this is so: AI requires new levels of performance in bandwidth, latency, and security. Further, as inferencing takes hold in companies, only the most efficient and top-performing networks can achieve the processing required to adapt AI models to fit a firm's specific requirements.
In Futuriom’s latest report, “What’s Next for Networking Infrastructure for AI,” we look closely at the evolution of networking for AI and the trends involved.
Trends in Networking for AI
Constructing networks for AI requires a revolutionary shift from legacy centralized datacenter computing to distributed, accelerated computing—an architecture that strings together up to thousands of GPUs and CPUs operating in parallel to comprise one big cluster, or to use NVIDIA's term, an AI factory.
To support networking for AI, existing technologies must be improved and new approaches created to sustain the performance demanded by AI applications. From rack to cluster to interconnected datacenters, AI requires a fresh take across all elements to ensure adequate performance.
This new focus starts within the rack, where advances in PCIe links between GPUs and CPUs and networking switches have been improved in speed and efficiency. There’s also innovation in the links between clusters, as Ethernet, streamlined for performance by the Ultra Ethernet Consortium (UEC), is helping to bring Ethernet on par with NVIDIA’s InfiniBand as a preferred scale-out networking protocol.
These are just a few of the trends afoot in networking for AI. Much more is happening, including a focus on specialized AI processors, optical components, and security.
Download the free report here now!
Special thanks to our Sponsors: Arrcus, Aryaka, DriveNets, and Versa Networks !
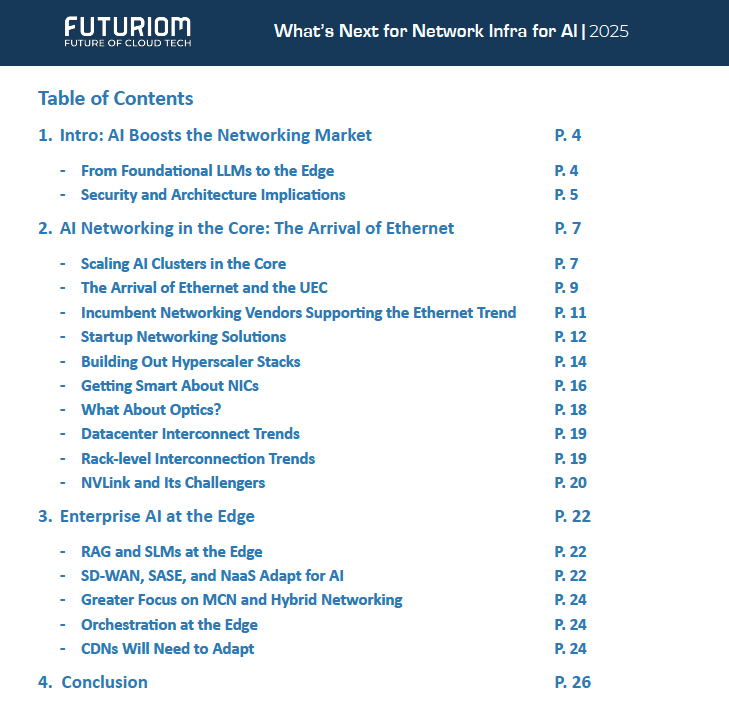
Highlights and Key Findings
- AI infrastructure needs continue to expand. Enterprises are starting to adapt large language models (LLMs) to fit their specific business requirements. A mixture of infrastructure will be needed to deliver LLMs for training, as well as specialized models including small language models (SLMs).
- Inferencing needs will expand infrastructure in a variety of ways. AI inferencing, which enables applications to take input and process output through AI models, will be distributed across cloud and enterprise infrastructure. As models evolve, more inferencing infrastructure will be needed to interpret and serve up results on a variety of devices and infrastructure from the data center to the edge.
- Ethernet is gaining ground against InfiniBand. Efforts to shift AI networks from reliance on NVIDIA’s proprietary InfiniBand networking technology is showing dramatic results, with adoption of Ethernet solutions being heralded even by NVIDIA as key to inferencing.
- The Ultra Ethernet Consortium remains relevant. Efforts by vendors, including NVIDIA, are coalescing around a standard that improves on Ethernet’s drawbacks and RDMA’s limitations.
- Speeds are increasing. While most AI datacenter switches support speeds of 400-Gb/s, 800-Gb/s rates are increasingly on the horizon, with even higher speeds in the works.
- Optical networking is part of AI’s future. As AI networks grow in scale, speed, and power requirements, optical components will furnish solutions that save power, space, and operational costs.
- AI specialized processors such as SmartNICs, IPUs, and DPUs are growing in importance for AI infrastructure. Network interface cards powered by specialized chips are key to enabling better performance of networking, security, and storage functions of AI networks. But switch vendors are finding ways to be “NIC agnostic” to avoid vendor lock-in.
- SASE, SD-WAN, and network-as-a-service will evolve to support AI networking with more pervasive security. AI will increase data traffic by orders of magnitude, but a distributed networking infrastructure is needed to connect these resources and provide enterprise controls and compliance.
- Observability and AIOps are central to AI networking ROI. The ability to track, analyze, and automate networking efficiency is becoming vital to enterprise adoption of AI networking.
- Companies included in this report: Akamai, AMD, Arista, Arrcus, Aryaka, Astera Labs, Aviz Networks
AWS, Broadcom, Ciena, Cisco, Cloudflare, CoreWeave, DriveNets, Enfabrica, Equinix, Google Cloud, Hedgehog, Infinera, Juniper Networks, Lambda Labs, Marvell, Meta, Microsoft, Napatech, Netris, NVIDIA, Oracle, Vapor IO, Versa Networks, xAI, ZEDEDA
Companies included in this report: Akamai, AMD, Arista, Arrcus, Aryaka, Astera Labs, Aviz Networks, AWS, Broadcom, Ciena, Cisco, Cloudflare, CoreWeave, DriveNets, Enfabrica, Equinix, Google Cloud, Infinera, Juniper Networks, Lambda Labs, Marvell, Meta, Microsoft, Napatech, Netris, NVIDIA, Oracle, Versa Networks, xAI, ZEDEDA