DriveNets Advances Its AI Networking
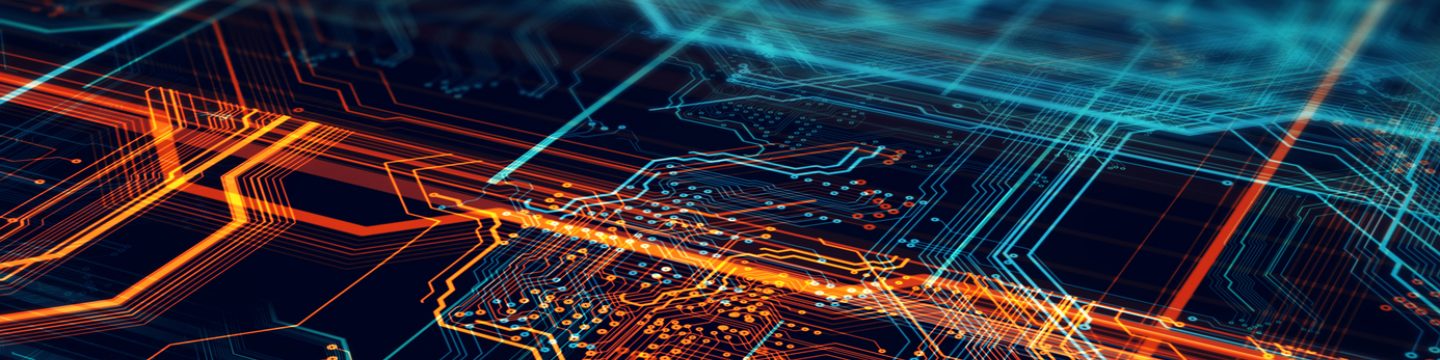
DriveNets has taken the wraps off a new version of its software designed to optimize networking for AI workloads. And the vendor has engaged independent testing to establish that its cell-based, scheduled fabric is faster, more reliable, and cost-effective than traditional Ethernet spine-and-leaf setups.
DriveNets’ Network Cloud-AI, initially announced in May 2023, is based on the Distributed Disaggregated Chassis (DDC) specification supported by the Open Compute Foundation (OCP). The OCP DDC offers an open architecture for a massively scalable software router that isn’t dependent on a single proprietary hardware chassis.
The first iteration of the DriveNets Network Cloud-AI series runs on white box servers based on the Jericho2C+ Ethernet switch ASIC from Broadcom, creating a distributed, cloud-based network for demanding generative AI workloads. This second release supports Broadcom’s new Jericho3-AI chip and Ramon 3 cell-based switching component, giving DriveNets’ DDC the ability to support 32,000 DPUs in a single cluster and up to 72 800-Gb/s Ethernet networking ports with load balancing and scheduling for deep AI training.
All in on Broadcom and Ramon 3
Related Articles
Google Bets Big on AI Infrastructure
Google parent Alphabet is spending big on AI infrastructure. But major progress in enterprise AI cloud isn't yet underway
Arrcus Lands $30 Million with Help from NVIDIAMCN software player Arrcus scores significant investment from NVIDIA, which is also collaborating with Arrcus on AI networking solutions
DriveNets Boosts Acacia Optical IntegrationDriveNets has added 400-Gb/s modules from Cisco subsidiary Acacia Communications to its disaggregated Network Cloud stack
DriveNets gives a few reasons why its architecture will be better for AI-based workloads. First of all, because it's based on Broadcom Jericho2C+ and whiteboxes, it delivers a scale-out architecture on industry standard whiteboxes, with the potential to lower capital spending (capex) costs. Scaling out the cloud-based systems is as simple as adding new whiteboxes.
The next advantage comes down to the Ethernet switching architecture, according to DriveNets. DriveNets says that using an Ethernet Clos architecture, which aggregates switching ports into a traditional networking backbone, can result in performance hits. Instead, it's using a cell-based scheduling technology cloud-based backbone. By adopting Ramon 3 cell-based scheduling, DriveNets says its fabric will perform better than traditional Ethernet fabric switches, with lower latency and jitter and better reliability. Further, Network Cloud-AI doesn’t need to be adjusted for specific large language models (LLMs), which DriveNets says can be an InfiniBand requirement.
Testing Results Back up Claim
DriveNets put these claims to the test by enlisting an independent datacenter simulation lab, Scala Computing, to set up a range of scenarios comparing Network Cloud-AI’s DDC with a leaf-spine Ethernet fabric. The results: DriveNets’ solution showed 10% to 30% improved job completion time (JCT) in a simulation of an AI training cluster with 2,000 GPUs. The improved JCT, DriveNets said, can lead to 100% system return on investment (ROI), since networking represents 10% of system cost.
The test lab also found that when running multiple AI jobs in parallel (as might happen in a multi-tenancy service), the DDC did not see a performance impact across all jobs when a “noisy neighbor” was introduced.
Any vendor-sponsored testing, of course, may cue the skeptics. But overall, the message is clear: DriveNets claims better performance and more efficient use of resources for AI workloads than proprietary Ethernet fabrics. And it avoids the kind of broad solution dependency associated with NVIDIA InfiniBand products.
Futuriom Take: DriveNets Network Cloud-AI software offers an Ethernet-compatible, cloud-based network that operates like a large, distributed switch backplane with cell-based scheduling. This different way of thinking will lead to some consideration about the networking architecture used to process AI workloads.
Related Articles
Aviz Follows Up Cisco Deal with $10 Million Round
Cisco joins a group of investors putting $10M into SONiC networking supplier Aviz Networks
DriveNets Boosts Acacia Optical IntegrationDriveNets has added 400-Gb/s modules from Cisco subsidiary Acacia Communications to its disaggregated Network Cloud stack
Futuriom 50: The 2023 StandoutsThe year's ending and Futuriom is starting to pick the Futuriom 50 list of top private companies in key markets for cloud and communications infrastructure.